Understanding Annotation in Machine Learning: A Comprehensive Guide
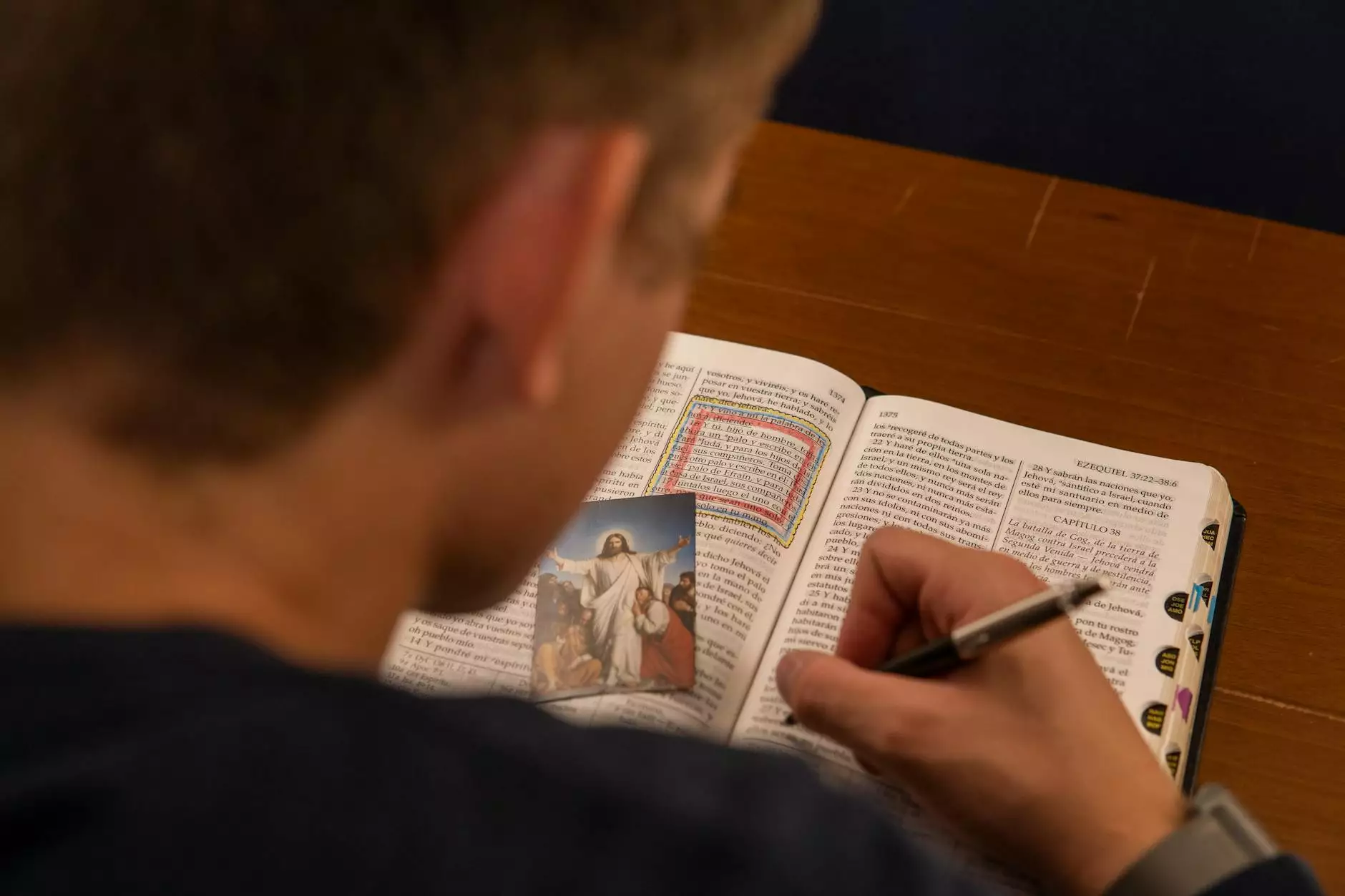
In the rapidly evolving landscape of technology, machine learning stands as a revolutionary force, transforming numerous industries, including home services like locksmithing. A critical element of machine learning is annotation. This article delves deeply into the concept of annotation in machine learning, its types, importance, and how it can significantly enhance business operations.
The Foundation of Machine Learning: What is Annotation?
At its core, annotation refers to the process of labeling data for machine learning models. The machines learn from this labeled data to make predictions or decisions without human intervention. The quality and accuracy of this annotated data play a crucial role in the effectiveness of machine learning algorithms.
Types of Annotation in Machine Learning
There are several types of annotation utilized in machine learning projects, each with its specific applications:
- Image Annotation: Labeling images with bounding boxes, polygons, or segmentation masks to identify objects.
- Text Annotation: Assigning tags to textual data, such as categorizing sentiment or identifying key phrases.
- Audio Annotation: Labeling audio clips for speech recognition or sound classification.
- Video Annotation: Marking video frames to identify moving objects or actions.
The Importance of Annotation in Machine Learning
Annotation is vital for several reasons:
- It provides context for the data, enabling the model to learn effectively.
- Accurate annotations improve the performance of the model, leading to better predictions.
- Customized annotations help in refining the models for specific business needs, such as in the locksmith industry.
How Annotation Impacts Business in Home Services
In industries such as home services, effective use of machine learning hinges on quality data annotation. For companies like KeyMakr, which specializes in locksmithing, annotated data can streamline operations and enhance customer service.
Consider the following applications of machine learning annotation in locksmithing:
- Customer Interaction: By analyzing customer queries through text annotation, locksmith companies can improve their response systems, anticipating customer needs more effectively.
- Service Dispatch: Using image and video annotation to train models that analyze the best routes and allocate resources efficiently during service calls.
- Fraud Detection: Implementing audio annotation to recognize patterns in voice calls, helping identify potential fraud scenarios.
The Process of Annotation in Machine Learning
To achieve high-quality annotations, it is essential to follow a systematic process:
- Data Collection: Gather the relevant data that needs to be annotated.
- Define Annotation Guidelines: Establish clear instructions on how to carry out the annotation.
- Select Annotation Tools: Use appropriate software or tools that facilitate the annotation process.
- Quality Control: Implement checks to ensure the annotations are accurate and consistent.
- Iterate and Improve: Collect feedback and refine the annotations and the overall process continuously.
Tools for Effective Annotation
Several tools and platforms can help businesses manage their annotation processes effectively:
- Labelbox: A robust platform offering tools for image, video, and text annotation.
- SuperAnnotate: A feature-rich tool designed for image annotation specifically for machine learning models.
- Amazon SageMaker Ground Truth: An integrated service that helps build highly accurate training datasets for machine learning.
- DataRobot: Offers automated data labeling features that simplify the annotation process.
Challenges in Data Annotation
While the benefits of data annotation are clear, businesses like KeyMakr may encounter several challenges:
- Scalability: As the amount of data grows, maintaining a consistent quality of annotations can be difficult.
- Cost: High-quality annotation processes may lead to increased operational costs.
- Time Consumption: Annotating large datasets can be time-consuming, delaying product rollouts.
- Human Error: Using human annotators can introduce biases and inaccuracies.
The Future of Annotation in Machine Learning
The landscape of machine learning is continually evolving, and so are the techniques for data annotation. Innovations such as semi-automated annotation and active learning are becoming increasingly common. These techniques can reduce the time and cost associated with manual annotation while maintaining or enhancing accuracy.
Automated Annotation
With advancements in artificial intelligence, automated annotation is gaining traction. By leveraging pre-trained models, businesses can automate parts of the annotation process, saving time and resources. However, human oversight remains essential to ensure quality.
Community-Based Annotation
Platforms that allow crowdsourcing for annotation tasks are also on the rise. These platforms enable a broader community to participate in the annotation process, potentially reducing costs and speeding up the process.
Conclusion
In summary, annotation in machine learning is a cornerstone of creating effective and efficient models. For businesses like KeyMakr operating in home services such as locksmithing, understanding and implementing robust annotation processes can lead to significant enhancements in operational efficiency and customer satisfaction.
As technology progresses, staying abreast of best practices in data annotation will ensure that businesses not only keep pace with the competition but also set the standard for excellence in their respective fields.
Call to Action
If you are involved in machine learning projects, consider investing in quality data annotation and explore how it can transform your business practices. Connect with experts to tailor these solutions to your home service needs.